Your all-in-one guide to marketing mix modeling (MMM)
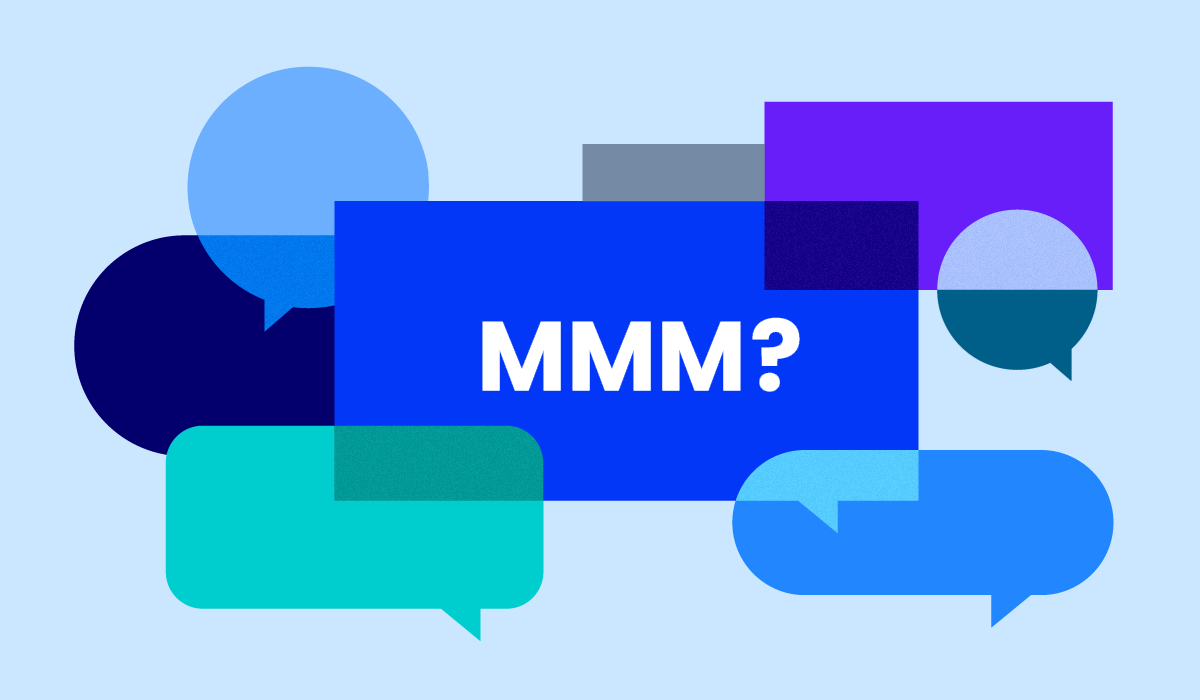
Necessity is the mother of invention. We all saw how COVID-19 had accelerated digital transformation over the past three years. Daily lives have been reorganized around social distancing measures, and digital processes have replaced more familiar and conventional ways of working.
The mobile marketing world is also going through a huge inevitable change. With privacy and security concerns increasing among users, we are forced to plan and optimize for the next normal. Thankfully, a new generation of marketing mix modeling (MMM) is expected to be a problem solver.
In this post, we will take a look at what MMM is, why it has come into the spotlight recently, how you should work with it, and everything else you need to know about it.
Before moving on, if you are confused about all the different mobile measurement acronyms – MMM, MTA, MMP, and more, check out the blog posts below.
👉 Why an MMP is an App Marketing Must-Have
👉 MMM vs. MTA: Which Is Right For You?
👉 Marketing Mix Modeling: The Privacy-First Mobile Measurement Method
MMM, which stands for marketing mix modeling or media mix modeling, is a statistical methodology used to estimate the impact of various marketing inputs on desired outcomes.
Using aggregated channel-level data as well as information about promotions, macroeconomic conditions, seasonality, etc., MMM allows you to answer questions like “What is the effect on sales of a search ads campaign in February compared to a display ads campaign in August?” or “What is the ROI of my print and TV spends?” The results are then adopted to adjust marketing tactics, forecast returns, and optimize future budgets.
Think of MMM as the process of understanding the effects of caffeine on sleep quality. Let’s take a look at the table below.
As you can see, sleep duration changes as caffeine intake varies, meaning there is a chance that these two are correlated. Likewise, MMM processes how each part of the marketing mix plays a role in driving sales and improving ROI. Some specific takeaways from this analogy are:
We know, MMM sounds like the ultimate measurement approach, and you might be wondering why you do not recall using it. In fact, MMM is making a comeback after being put on the back burner.
The term “marketing mix” was coined in 1949 by Neil Borden. A professor of advertising, he stated in his 1964 journal article, “When building a marketing program to fit the needs of his firm, the marketing manager has to weigh the behavioral forces and then juggle marketing elements in his mix with a keen eye on the resources with which he has to work.”
Over time, many other scholars added breadth and depth to the concept and MMM was one of the few tools available for holistic measurement before the digital age. Kraft Foods, an early user of this analysis, monitored whether their sales increased by promoting Jell-O on different television networks and magazines in different regions at different times of the year.
Nonetheless, the use of MMM was rather limited in the 20th century. Only the largest consumer goods companies could implement it because they were the first to have access to relatively accurate data on marketing, sales, and pricing. In addition, marketers were constantly challenged to optimize their marketing mix, but traditional MMM did not provide day-to-day results. One might say it was not surprising to see attribution modeling become the go-to approach for marketing measurement when real-time user-level tracking became available.
It seemed like MMM was falling powerlessly. However, the looming demise of third-party cookies and recent introduction of tighter privacy regulations made a turning point. Today, MMM has risen an essential part of the marketing survival kit for better decision making in this privacy-first era. Many tech companies reinvented MMM as SaaS solutions, opening doors to speed, scalability, cost effectiveness, and most importantly, inclusivity.
As the latest technological advancements have made MMM much more affordable and accessible, we suggest working with martech companies like Airbridge and fully enjoy the benefits of using SaaS MMM. However, if you have already decided to get full-suite consulting service or to try building internal MMM capabilities, here are some steps you should know.
There are many ways to build a model including the Bayesian approach, Shapley Value Regression, and tree-based ensemble machine learning. However, the most popular is multivariate regression due to easier model interpretability.
While regression models in general “fit a line” to the observed data to describe relationships between variables, multivariate regression is used to estimate (i) the strength of the relationship between two or more independent variables and one dependent variable and (ii) the value of the dependent variable at a certain value of the independent variables. The equation can be either linear or non-linear, meaning that some marketing inputs are directly proportional to the increase in sales while others are not.
Another important point is that MMM uses multivariate regression to analyze time series data, which helps with understanding trends and patterns over time. Time series data is collected at regular intervals, such as daily, weekly, or monthly and can be used to forecast future values of a variable based on past values. This is how MMM not only examines the past but also predicts the future.
Any statistical modeling starts with selecting appropriate variables to find a good model from a set of possible models.
Usually in MMM, the dependent variable is business performance, such as sales, revenue, market share. It can be further broken down into base outcome, which is the natural demand for the product driven by pricing, seasonality, brand equity and brand reputation, and incremental outcome, which is produced as a result of marketing activities. This data should be collected over the same period of time as the ad spend data.
Common independent variables include the following.
Note that variable selection can be very complex because you need to sort out and leave only the factors that actually affect your performance. Moreover, in the real business environment, multiple marketing campaigns tend to happen at the same time. This leads to multicollinearity, which occurs when two or more independent variables in a regression model are highly correlated with one another. This is not a problem per se, but it makes it difficult to determine the unique effect of each independent variable on the dependent variable.
Multicollinearity can be detected by using statistical methods such as correlation matrix and Variance Inflation Factor (VIF). To fix multicollinearity, you can remove one or more correlated independent variables from the model, combine correlated independent variables into a single composite variable, or collect more data to reduce the correlation among independent variables.
MMM is a powerful tool, but like any tool, it has its limitations that you should be aware of. First and foremost, MMM does not establish causality. It only demonstrates correlations between variables, meaning that it does not prove that a business outcome is the consequence of a certain marketing activity or ad spend. This also implies that MMM relies on certain assumptions about the data such as linearity and inalterability. If the assumptions are not met, the analysis results may be biased.
Second, MMM does not capture marketing performance in the most precise manner. Using aggregate data can make it difficult to see the impact of specific media tactics or channels. In addition, the aforementioned multicollinearity can pose challenges to determining the unique effect of each independent variable on the dependent variable.
Third, MMM may not be the most agile measurement approach you can find in the market. Preparing months worth of data and training the model with it takes time, and in the worst case scenario, if the data is of poor quality, the analysis may be limited. It should also be noted that the norm is to update the model on a quarterly or semi-annual basis, thus making it difficult for marketers to respond to the rapidly changing market situations.
Of course, there are alternatives. Airbridge MMM, for instance, auto-collects your performance and cost data and provides several types of reports that are updated every day or every week. Nonetheless, we recommend that you use it in conjunction with other measurement approaches like last-touch attribution and multi-touch attribution to draw a comprehensive conclusion.
Phew, we say this is enough for now! We touched upon a lot of different concepts, so be proud of yourself for reading till the end.
Key takeaways of the day:
If you do not want to miss the latest updates about MMM, stay tuned for our upcoming posts. We are more than excited to share insights and experiences we have acquired as a mobile measurement partner with powerful MMM features!